Alert fatigue and cyber staffing shortfalls continue to challenge security teams, especially as nation-state cyber attacks, which have doubled over three years, keep analysts on constant alert. The Russian attack on Ukraine has made matters worse as SOC teams from all sectors, especially energy and finance, are being called on for increased vigilance. Even unsophisticated attacks that nevertheless can wreak major damage are on the rise. McKinsey recently reported “a near-sevenfold increase in spear-phishing attacks” since the pandemic began.
Security operations teams simply cannot keep up. Sixty percent of enterprise-level SOC analysts can triage only less than 40% of log data (McKinsey). This is a function of both the complexity of modern IT systems, which include hybrid on-prem and cloud, each with targeted security coverage, and the relative rarity and high cost of trained security professionals with the skills to triage and investigate across all these ecosystems and tools. Malicious threats are going undetected and/or un-investigated. Indeed, visibility is limited in the murky waters of the vast cyber sea.
Fortunately, we can look to teams armed with a new way to think about artificial intelligence for cybersecurity to solve this problem.
Moving past "one-off analytics"
Modern data processing systems are based on a hierarchy of sequential processing steps. One-off or single-use analytics can be used for occasional needs, but for embedded, real-time analytics, one must automate a series of successively more sophisticated processing steps, each of which builds on the step that precedes it. For image recognition, this means detecting edges and colors first, then shapes, then object, and only then classifying the image. For security, this means starting with knowns before proceeding to unknowns.
The knowns of cybersecurity are signatures, or IOCs. Signature-based alerts are table-stakes that also have a relatively low false positive rate. However, they are static and reactive, and therefore have low efficacy for new or evolving threats.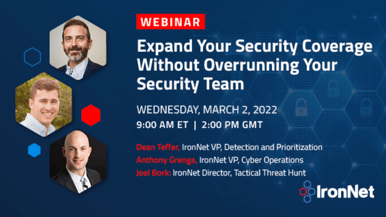
The next level is behavioral detections. These are necessarily based on more data, such as history, statistical measures, and lower-level signatures or “factual” events. Unlike signatures, behavioral detections can identify novel events and new attack types or events that vary by situation or configuration. They are relatively high false positive, however.
The next frontier in security builds upon the prior levels by automatically correlating signature and behavioral detections. These can yield dynamic, novel, and situational detections, but with relatively low false positives. In addition, since all available relevant information is automatically collated in advance, these alerts provide actionably content, unlike the simpler events produced by signature and behavioral detection.
“CODE-ifying” human threat intelligence
As one who lives and breathes in the world of data analytics, I will continue to shout from the rooftops that analytics without human intelligence will never cut through the cacophonous noise that every SOC analyst is forced to endure. Why not? It’s simple: their tools are missing the “So what?” that can calm even the nosiest of SOCs.
The secret ingredient here is human smarts. In the case of IronNet, our automatically correlated alerts are infused with the cumulative human intelligence of elite, Tier 3 analysts and threat hunters, allowing us first to fine-tune the detections themselves and, second, pre-package corroborating investigation information with the automated alerts. In other words, we have “CODE-ified” human intelligence to allow analysts to prioritize actionable alerts and pivot quickly to triage.
Leveraging human insights advances the power of analytics, turning the messy reality of SOC detections to more predictable, relevant, and actionable threat alerts.
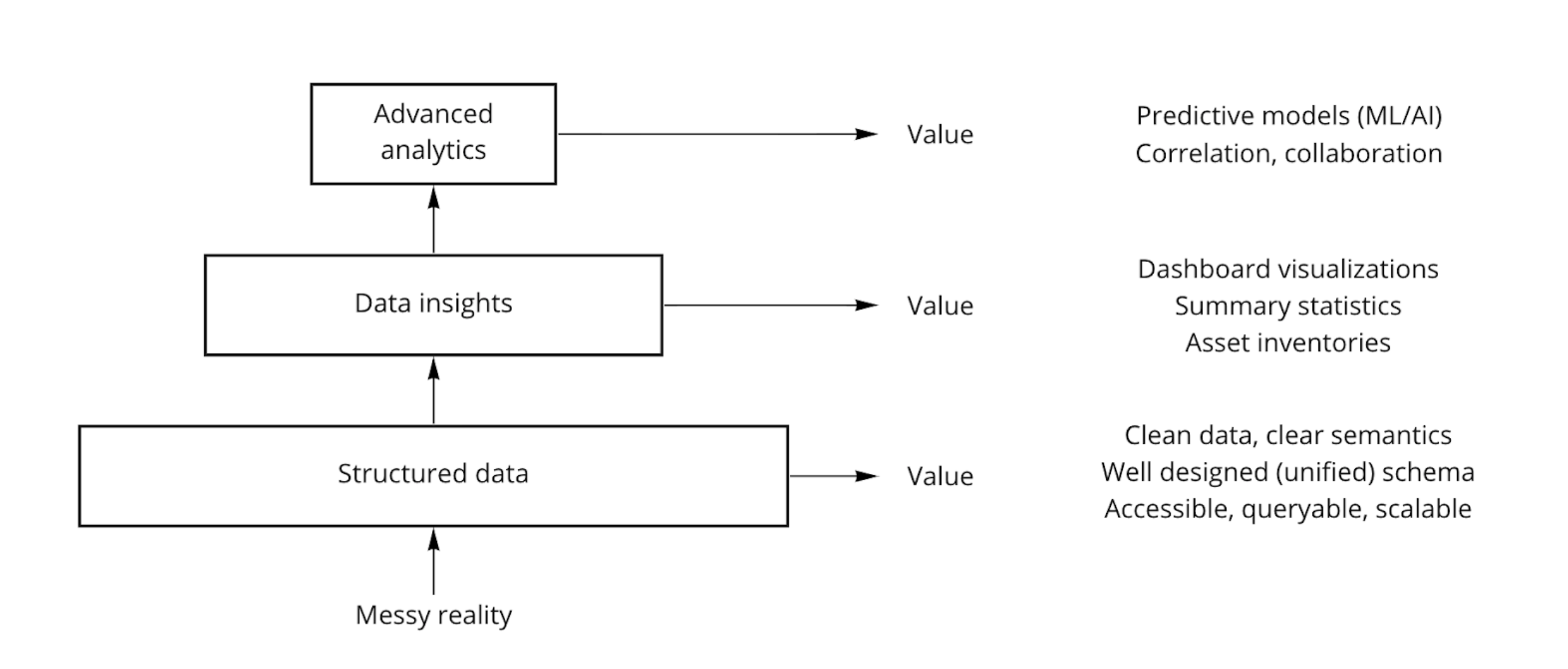
Join me and my IronNet threat intelligence colleagues this Wed., March 2, 9:00. Click here to register.
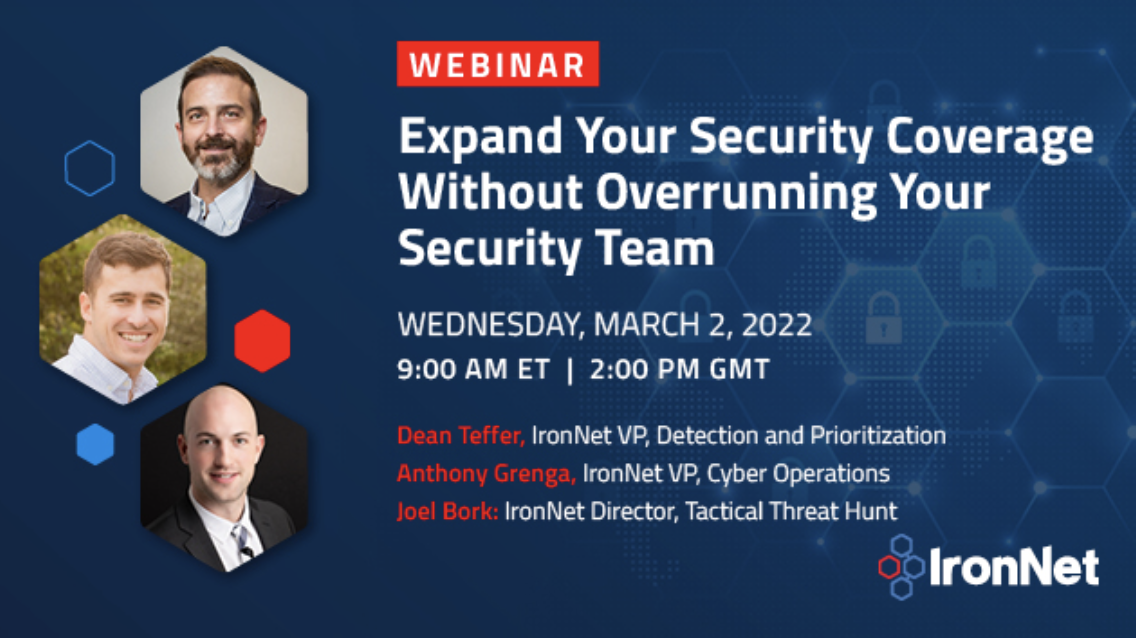