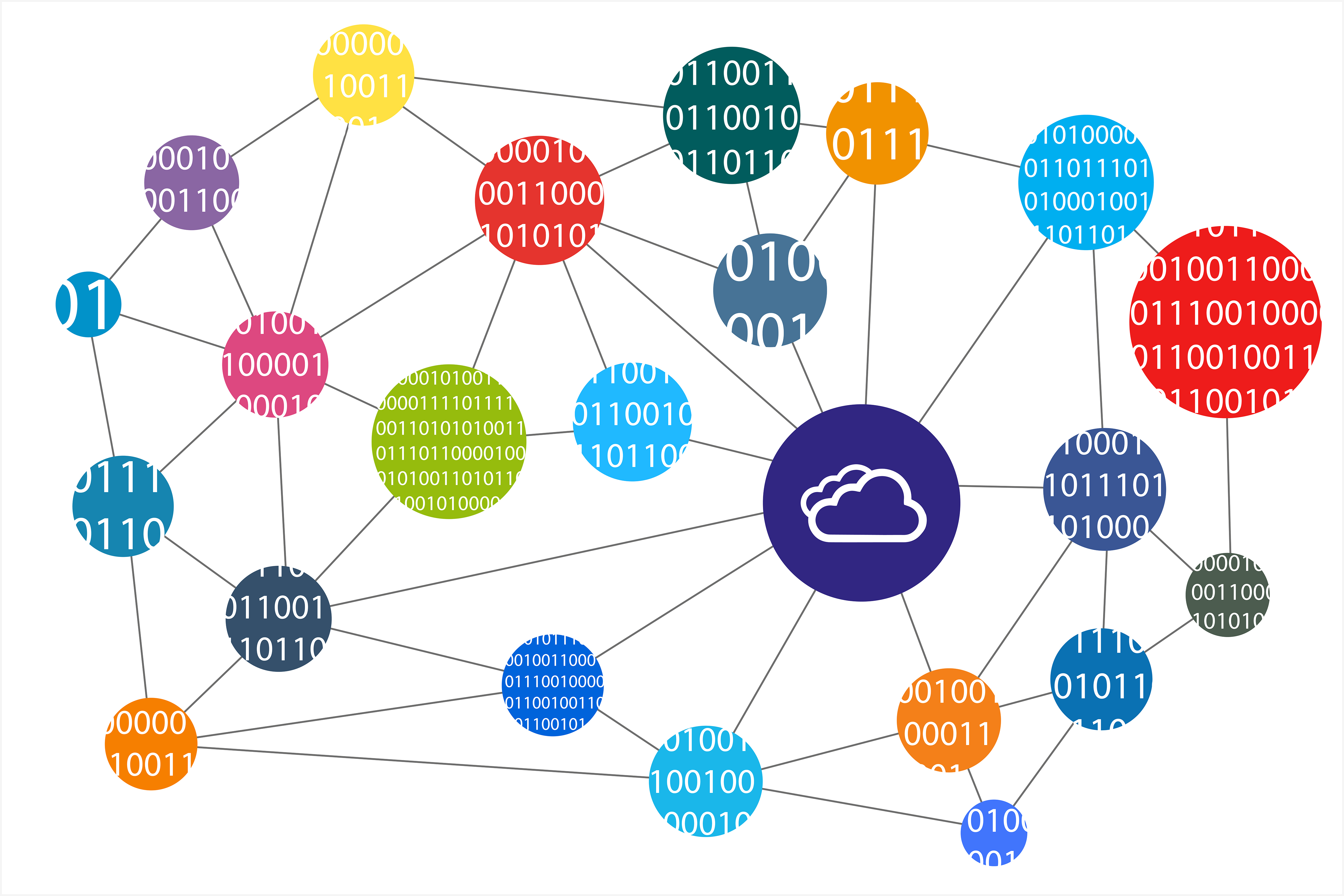
Alert fatigue and staffing shortfalls have been two of the most-commonly cited issues facing security team managers and members for many years. An increasingly large ecosystem of products has made little dent in this situation. In fact, installing more monitoring products generally yields more alerts for review. Jamming all the alerts into a SIEM can increase convenience for the operator and hunter, but doing so does not necessarily enable more efficient hunting or query across all these sources. Add in cloud-native data, which has increased significantly in volume and importance during COVID-19, and this situation only continues to become more acute.
Why does machine learning and algorithmic threat detection still yield false positives? After all, we experience high-quality algorithmic results every day using natural language processing and image processing. Why not threat detection?
There are two primary reasons false positives remain a thorn of discontent for most SOC analysts:
This might be a shocking statement to make in light of the SolarWinds attack, the Microsoft Exchange server hack, and the ransomware attack on Colonial Pipeline, but what this means in average times is that a detector that always yields a “no threat” label would be accurate almost all the time. In order to (sort of) turn up the gain on the detector to make sure we do not miss any potential threat event, we necessarily incorrectly label some events as “threat” when they should not be.
Even if we do tune well for both detection of all actual threat events (recall) and simultaneous minimization of false detection (precision), unlike image recognition or text, an apple is not always an apple in security. Outside the narrow scope of signature-based detection, behavioral anomalies and threat signals are manifest in real-work networks in a myriad of ways, which frequently vary based on specific configurations of infrastructure, IT policy, and user conventions.
A practical way to reduce false positives
Fortunately, there is an activity that is both essential to threat hunting and directly in support of false-positive reduction: identifying corroborating evidence. This is, in fact, one of the primary activities of the threat hunter and SOC operator. However, an alert that fires on a network log, indexed by ip address, is difficult to correlate to information in the Active Directory log, let alone the AWS security log. This is why it takes so long, and why the activity requires so much expertise that, as already mentioned, is difficult to recruit.
What if all data were tagged on ingest with a device or user id, regardless of data source, and any information across the ecosystem related to entity association (user authorization, device registration, etc). were tracked and recorded, so that ALL events detected on a device or associated with a user could be not just searched but also automatically combined? This would, in fact, be the set of corroborating evidence a threat hunter is trying to assemble. Also, such a set of events could inform a model-driven Bayesian conditional probability of the likelihood that not just one event but a whole sequence of events, comprising, say, three distinct MITRE ATT&CK stages, has been detected within the past two days. Such a likelihood function would yield a more robust and mathematically defensible measure of severity and confidence.
Correlating detected network threats
The correlation of detection analytics is one thing. Behavioral analytics enriched by human insights is another. This scenario gets us closer and closer to minimizing false positives and reducing the margin of error. That is the goal of the Expert System in IronNet's IronDefense NDR solution. But correlation across SOC analyst teams in a Collective Defense ecosystem drives home the difference between crying wolf and an urgent and real need to batten the hatches against the real wolves lurking the network.
IronDefense correlation engine
Threat analysts and hunters spend a significant portion of their time triaging individual alerts by identifying corroborating evidence and related information. IronNet is currently working on a new correlation engine that models adversary attack techniques and pre-correlates anomalous activity by threat categories to improve risk scoring and alert prioritization, in turn dramatically reducing alert load.
This system leverages a multi-pass system that first optimizes for detecting as many potential instances of a particular type of threat activity and enriching detections with threat intel and other external and internal data sources to optimize for detection precision. Events are further aggregated by entity information, attack stage identification, and time sequence data to deliver a timeline of an attack and scored by risk to the enterprise.
IronNet’s goal is to help SOC analysts do what they do but even better. By using behavioral analytics to make existing tools smarter by focusing on unknown threats that signature detection often misses, security analysts can rely on IronNet’s correlation engine, along with real-time threat sharing in a Collective Defense community, to help overcome the curse of too many false positives.